Ever wondered what actually happens behind the scenes when you hit “Translate” in an app or website?
AI translation tools seem almost magical. They turn content from source text into another language in seconds. But behind that quick result is a lot of clever technology doing the heavy lifting.
📚 Jargon-free guide
In this article, we’ll break down how AI translation works in simple, human language. This way, you can understand what’s happening behind the scenes and why it matters when you’re scaling content across languages
What is AI translation?
AI translation is the use of artificial intelligence to automatically convert text or speech from one language to another.
Instead of translating text word by word, AI translation tools look at the bigger picture. They analyze the entire sentence (sometimes even the whole paragraph) to figure out what’s actually being said and how it should sound in another language. Translations generated with the help of AI are typically more accurate than what rule-based or word-for-word systems offer.
🤖 Machine translation vs. AI translation
Let’s take a quick look at the difference between machine translation and AI translation. Machine translation systems rely on fixed rules and dictionaries, whereas AI translation tools use advanced algorithms like neural networks. This is how they’re capable of quickly understanding context, meaning, and structure of the source language.
How AI translation works
AI translation works by using artificial intelligence to read a sentence, understand what it means, and then rewrite that meaning in another language. There’s a lot of sophisticated technology making that happen.
Let’s break it down in a way that’s easy to understand.
Step 1: The model learns languages
AI translation starts with training a model. Imagine you’re teaching someone to become fluent in multiple languages, but not by explaining grammar rules. Instead, you’re showing them millions of real-world examples. That’s exactly what happens.
The model is fed massive amounts of bilingual or multilingual data. Think websites, books, subtitles. This is how it learns patterns, sentence structures, and vocabulary across different languages.
Over time, the model doesn’t just memorize word pairs, but also starts to understand how meaning is shaped by context, word order, and nuance.
Step 2: Turning words into numbers
Here’s where the AI part kicks in. The model doesn’t “see” words the way we do. It converts each word (and sometimes each character) into a vector. A vector is a long string of numbers that capture the meaning and relationship between words. This is called embedding.
For example, in English, the words “cat” and “kitten” will have similar vectors because they’re related. The same goes for their equivalents in other languages.
Step 3: Encoding the sentence
Once the words are turned into numbers, the model runs them through a thing called an encoder. You can think of this as the part of the system that reads and understands the source sentence. It looks at the entire sentence, not just word by word, and creates a kind of compressed summary of its meaning.
Step 4: Decoding into another language
Then comes the decoder. This is where the magic of translation occurs. The decoder takes the compressed summary and starts rebuilding the sentence in the target language. It does so by looking at the entire meaning and predicts the next best word, one by one, until the sentence is complete.
Step 5: Adding context with an attention mechanism
To make translations more accurate, modern systems use something called an attention mechanism. Think of it like a spotlight that helps the model focus on the most important words or phrases in the original sentence while it’s translating. This is why AI translations today are much better at handling longer, more complex sentences than older machine translation systems.
Step 6: Continuous learning
AI translation models don’t stop learning once they’re built. They continue to improve after they’re launched by analyzing new data, spotting mistakes, and adjusting their translations. For example, if enough people correct the same translation over and over, the model can learn from those corrections and avoid making the same mistake next time.
AI translation use cases and tools
AI translation is used to localize products and apps, adapt e-commerce content, support multilingual customer service, and speed up global marketing and content creation. Let’s take a closer look at the use cases and most popular tools.
Product and app localization
Translating user interfaces, feature updates, and in-app messages used to take weeks. With AI, teams can localize product content quickly and keep releases on schedule. It’s especially useful when you’re supporting multiple markets at once.
E-commerce
AI translation helps online retailers adapt product descriptions, reviews, banners, and checkout flows for global audiences. It’s fast and scalable, perfect for teams managing thousands of SKUs across multiple regions.
💳 Want to learn more about e-commerce localization?
Read this comprehensive guide on how to translate your Shopify store with ease.
Customer support
AI translation is used to translate live chat responses, knowledge base articles, and help center content. This helps support teams respond faster and serve users in their native language (even without hiring native-speaking agents for every market).
Marketing content
From blog posts to email campaigns, AI translation gives marketing teams a strong first draft they can polish and publish faster. It’s especially useful when working on multilingual launches or seasonal campaigns under tight deadlines.
📚 Further reading
Wondering which tool to use? Side by side with native speakers, we tested the most popular ones based on accuracy, speed, cost, and ease, and highlighted important factors to consider. Discover the best AI translation tools on the market.
How AI translation models are trained
Training an AI translation model is a little like teaching someone a new language, but on a much bigger scale (and much faster). The process goes like this:
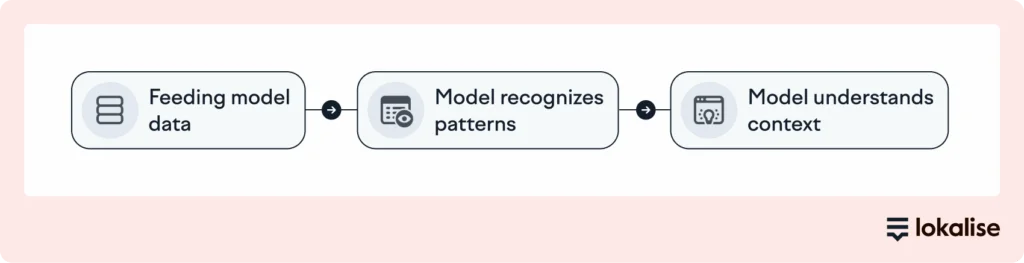
It all starts with data. Lots of it. AI models learn by analyzing millions (sometimes billions) of sentence pairs in different languages. These pairs are examples of the same sentence written in two languages. For example, it could be English and Spanish versions of a news article.
The model is then “fed” huge bilingual datasets. Everything from subtitles and website translations to international news articles, and other real-world content where the same message appears in multiple languages.
Gradually, the model starts noticing patterns. This helps it learn how words, grammar, and meaning shift from one language to another.
With time, the model learns to spot context, tone, and word order so that it can make the translation sound natural, not robotic.
How did AI translation evolve?
AI translation didn’t appear overnight. It’s the result of decades of progress, with each stage making translations faster, smarter, and more accurate.
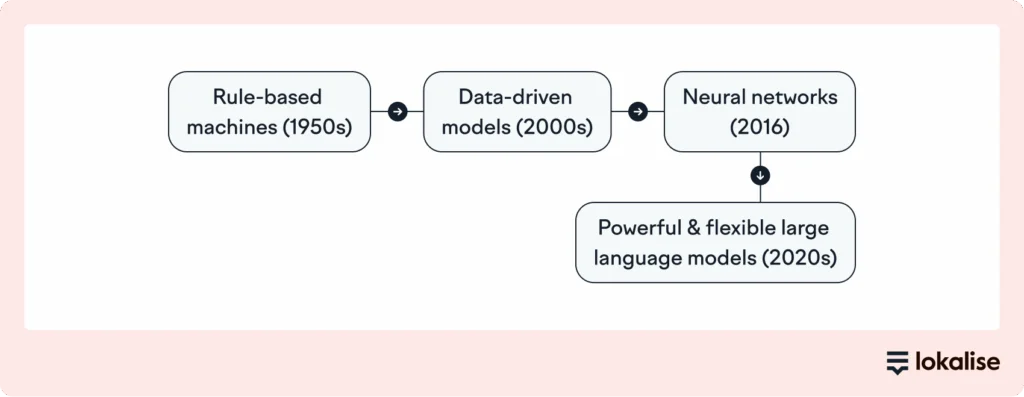
1. Rule-based machine translation (RBMT) — 1950s-2000s
In the early days, translation tools worked by following strict grammar rules and bilingual dictionaries. These systems were built by linguists who manually taught machines how to translate. But language isn’t always that simple, so the translations often felt clunky and literal.
2. Statistical machine translation (SMT) — 2000s-2010s
In the 2000s, statistical models took over. Instead of relying on fixed rules, these systems used huge bilingual datasets to figure out which words were most likely to match. It was all about probabilities and data patterns. This made translations a bit smoother, but they still struggled with long sentences and nuance.
3. Neural machine translation (NMT) — 2016 and beyond
The real breakthrough came around 2016. That’s when neural networks started being used in translation. NMT systems could look at entire sentences at once, understand the context, and produce more natural-sounding translations. One of the best known machine translation software, Google Translate, switched to NMT in 2016. The difference was noticeable overnight.
🧠 Google’s zero-shot translation
In 2016, Google introduced a new AI translation breakthrough called zero-shot translation. Simply put, it means the system could translate between two languages even if it had never seen that specific language pair during training.
For example, it could translate directly from Japanese to Korean without needing to first go through English. This was possible because Google’s multilingual neural machine translation system started to “understand” the meaning behind sentences in a more language-agnostic way (sort of like learning an internal universal language).
4. Large language models (LLMs) — 2020s and beyond
Today, AI translation is entering a new phase with large language models like GPT and others. These models can rewrite, adapt tone, and make translated content sound human. They’ve been trained on massive multilingual datasets, which makes them better at handling slang and cultural nuances.
Ethical concerns and AI translation
Ethical concerns in AI translation include issues like bias in language, loss of cultural nuance, data privacy risks, and the potential impact on human translators.
One key thing to bear in mind about AI is that it’s heavily reliant on the input.
The data used to train an AI model might contain stereotypes, biased language, or cultural inaccuracies. The model can then reproduce and amplify those same issues when translating text. This can lead to mistranslations, unintended offense, or reinforce harmful assumptions, without people even realizing.
🔒 Are AI translation tools secure?
The question of AI security is an important one. Some translation tools process large volumes of personal or confidential data, which raises privacy and security concerns if that data is stored, reused, or shared without consent.
To prevent any security issues, make sure you use trusted providers that are transparent about how they handle your data. Limit what you send for translation and never share confidential information (e.g., sensitive business data, personal details).
Where is AI translation heading?
AI translation is quickly evolving toward more accurate, personalized, and ethical solutions. You can expect:
- Better context understanding
- Fewer human edits needed
- More accurate and on-brand translations
Today’s AI translation models are great at sentence-level translation, but they can still miss the bigger picture. Future models will get better at handling context beyond individual sentences, which will improve flow, consistency, and accuracy.
AI translation will keep getting closer to human quality. We’ll likely reach content quality that requires little to no post-editing. This will save localization teams even more time and resources, especially when dealing with large volumes of content.
Because of cost-efficiency and all the hard-to-deny benefits, AI localization will become more prominent by default.
Lastly, it’s likely we’ll see more fine-tuned models trained for specific industries (e.g., legal, medical, or SaaS), and even tailored to individual brands. This will allow companies to maintain their unique voice and terminology, even without human input.
However, looping in a human translator is a responsible thing to do, even if it’s just for post-editing and quality assurance.
🧠 Did you know?
You can give AI translation tools more context by using translation memories, glossaries, style guides, and visual references. This data helps them better understand meaning, tone, and how the content will be used.
Want to see how AI translation works?
You can use AI to translate everything from websites and product descriptions to customer support chats and mobile apps. If you’re curious to see it in action, we invite you to check out Lokalise AI.
Lokalise AI helps you translate content up to 10× faster and cut translation costs by up to 80%, without compromising on quality. The tool is an integral part of a comprehensive localization platform.
It combines machine translation with your existing translation memory, glossaries, and brand guidelines, so that you get accurate, on-brand translations right from the start.
Plus, Lokalise AI is context-aware, meaning it can adapt to your industry, tone, and content type, making the output more natural and reliable.
What do give it a go? Explore Lokalise AI today.