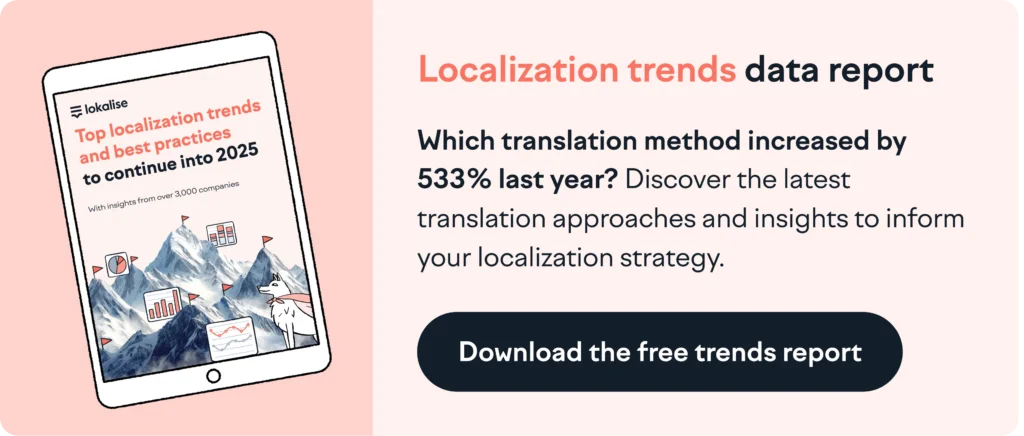
DeepL and Google Translate are probably the first tools that spring to mind when you think of translation technology, but the landscape is a lot more diverse than machine translation tools.
❓ Wait, so what is translation technology?
Translation technology includes everything from the tools that translate text from one language to another, the software used to manage translations and multilingual content, and, more recently, the AI-driven tools that generate multilingual content.
Before looking at the current translation tech landscape and predictions for the future, we’ll take you on a quick journey through the history of translation software. But first, let’s take a look at everything the translation tech landscape has to offer.
What falls under the translation technology umbrella?
As mentioned above, the language tech landscape is diverse and, with the rise of GenAI, new technologies are emerging weekly. Below, we’ve covered some of the main categories, taking inspiration from Nimdzi’s Language Technology Atlas.
The top 6 tools in the translation tech stack
- Machine translation tools
These include traditional machine translation tools like DeepL and Google translate and newer GenAI translation tools, powered by large language models like Open AI, Gemini, and Claude.
🤖 A quick word on AI
AI stands for artificial intelligence, a term that has been around for some time. AI translation and machine translation are often used interchangeably. But, now, AI is also being used to describe generative AI translation.
So, to avoid further confusion, we’ll refer to tools that translate with generative AI as generative AI tools (GenAI). Not AI translation or machine translation tools.
If you’re curious about how AI translation works, read the linked jargon-free guide.
- Translation management systems
Translation management systems (TMS) are exactly what they say they are. They are used to manage translations to make the process easier and faster. Apart from some nifty project management features, they have built-in machine translation capabilities, and easy-to-set-up automations that reduce manual work. Some also let you hire professional translators directly through their platforms. What’s more, they often have core linguistic assets and processes built-in, including:
- Translation memory
- Glossary
- Quality assurance (QA)
- Style guide
- CAT: Computer-assisted translation
An oldie but a goldie for linguists, CAT tools are computer-assisted translation tools that help translators improve consistency and speed up their projects. Like Grammarly, CAT tools suggest spelling corrections and syntax suggestions. They differ from machine translation because they use translation memory (TM) to store and suggest previously translated phrases, helping translators work more efficiently.
- Audiovisual translation tools
While watching some of your favorite non-native movies or series, you may have noticed the exceptional translation and dubbing quality. Behind these companies are tools that make it easy to translate sound and visuals, from project and asset management tools to AI-enhanced dubbing tools.
- Multilingual content generators
Since the rise of generative AI, players like Jasper, Copy.ai, and ChatGPT have implemented multilingual content generation into their offerings. Instead of copying and pasting the original version, you can create multilingual content from the get-go using multilingual content generators. Here’s an example of how ChatGPT writes an email in Spanish from scratch:
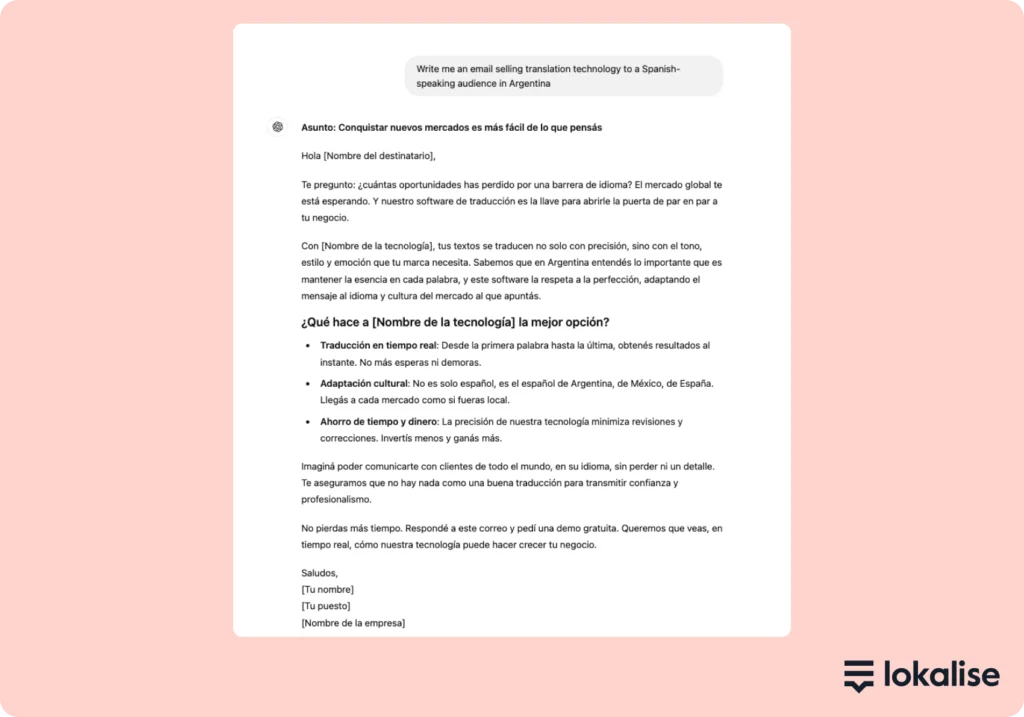
- Interpreting systems
After the Nuremberg trials in 1945, simultaneous interpreting became more commonplace than consecutive interpreting (where the interpreter starts to interpret after the speaker pauses). It saved a lot of time and was delivered and facilitated using an electronic interpreting system. Today there’s plenty of software that makes simultaneous interpreting easy, whether via video or over the phone.
💡Key takeaway
In short, translation technology is any software that aims to facilitate multilingual content translation and management without compromising quality, to make sure that millions of customer experiences are, to quote Netflix, “nothing short of exceptional”.
A brief history of translation technology
The first attempt at machine translation was in 1954, when an IBM 701 computer automatically translated a handful of carefully picked sentences, proving that machine translation was possible.
Despite the time-consuming research, considerable expenses, and limitations (the machine could only translate 250 words), IBM’s translation tech paved the way for future iterations. Though, we’d have to wait another 40 years or so until translation technology was readily available to the masses.
Did you know?
At the end of the 1950s, the US government was already looking into the possibility of fully automatic high-quality translation by machines. Only now are we coming close to this reality.
The evolution of translation technology since 1954
1954: The Georgetown-IBM Experiment marked the first time machine translation was demonstrated in public, Though it could only handle 250 words and struggled with basic translations, it sparked worldwide interest in translation technology.
1960s: The US government invested heavily in MT research through the Automatic Language Processing Advisory Committee (ALPAC). However, in a 1966 report, they concluded machine translation was more expensive, less accurate, and slower than human translation, which led to reduced funding and a period known as the ‘AI winter.’
1970s: The US Department of Defense and DARPA began developing speech recognition technology. This decade also saw the emergence of rule-based machine translation (RBMT) systems, which used linguistic rules to analyze and translate text.
1978: SYSTRAN, one of the oldest machine translation companies founded in 1968, started developing an English-to-French machine translation system in February 1976. In mid-1977 work began on the creation of the French-to-English system, and, in 1978, the company delivered its first MT system to the European Commission, marking the beginning of MT use in major international organizations.
Early 1980s: The first electronic dictionaries appeared, revolutionizing how translators accessed terminology. One of the most important projects was the EUROTRA project, launched by the European Community to produce a large grammar and general language dictionary, as well as a term dictionary.
1984: The ALP System from Coventry Lanchester Polytechnic University emerged as one of the first translation management systems, though primitive by today’s standards.
1988-1993: IBM researchers at the Thomas J. Watson Research Center introduced and developed Statistical Machine Translation (SMT) through their increasingly sophisticated models (IBM Models 1-5). This marked a shift from rule-based translation to probability-based approaches and led to the first word-based SMT systems that analyzed how individual words translated between languages.
1992: TRADOS released the first commercial Translation Memory system, MultiTerm, transforming how translators could reuse previous translations.
1993-1995: CAT (computer-assisted translation) tools began to appear with several key players entering the market:
- 1993: STAR Transit
- 1994: Déjà Vu
- 1995: OmegaT (one of the first open-source CAT tools)
1997: IBM introduced phrase-based statistical machine translation, improving upon word-based systems by considering groups of words and their context rather than isolated words. This became the dominant approach to machine translation for the next two decades until Google Translate switched from SMT to Neural Machine Translation (NMT).
2000-2002: The first generation of cloud-based Translation Management Systems appeared, with XTRF (2000) and Across Systems (2002) leading the way.
2006: Google Translate launched, using statistical machine translation to become the most widely-used translation tool globally. The system initially translated target languages through English as an intermediate language.
2010: Moses, an open-source statistical machine translation system, gained popularity among language service providers wanting to build their own MT engines.
2015: This year marks the beginning of the Neural Machine Translation (NMT) era, and a major shift in the industry. Neural Machine Translation began emerging as a viable technology, with early adopters experimenting with its potential.
2016: Google Translate switched to neural machine translation, dramatically improving translation quality and sparking an industry-wide shift toward NMT technology.
2017-2019: Major developments in NMT:
- 2017: DeepL launched, setting new quality standards for neural MT
- 2018: The first adaptive neural MT systems appeared, learning from post-editing corrections
- 2019: Transformer-based models revolutionized MT quality
2020-Present: Finally, enter the machine learning and GenAI era, which has led to context-aware translation systems that can better understand nuance and cultural context. Modern TMS platforms and other translation technology have also benefited from the rise of GenAI, adding AI-powered quality assessment, automated project management, and real-time collaboration features to their portfolio.
Translation technology today: it’s all about GenAI
Today, it’s almost hard to have a conversation without mentioning the word, AI.
Whether it’s to talk about content creation with AI or using AI as a personal assistant to do the jobs you hate, there are a lot of thoughts and mixed feelings.
One thing is certain though: AI is here to stay. And it’s impacting the translation industry in ways many thought were impossible just a few years ago.
When generative AI translation tools first hit the scene, there was a lot of skepticism around accuracy. They were seen as just another machine translation (MT) tool — tools like Google Translate and DeepL.
Last year, we sent a survey to 13,000 Lokalise customers that reflected this perception:
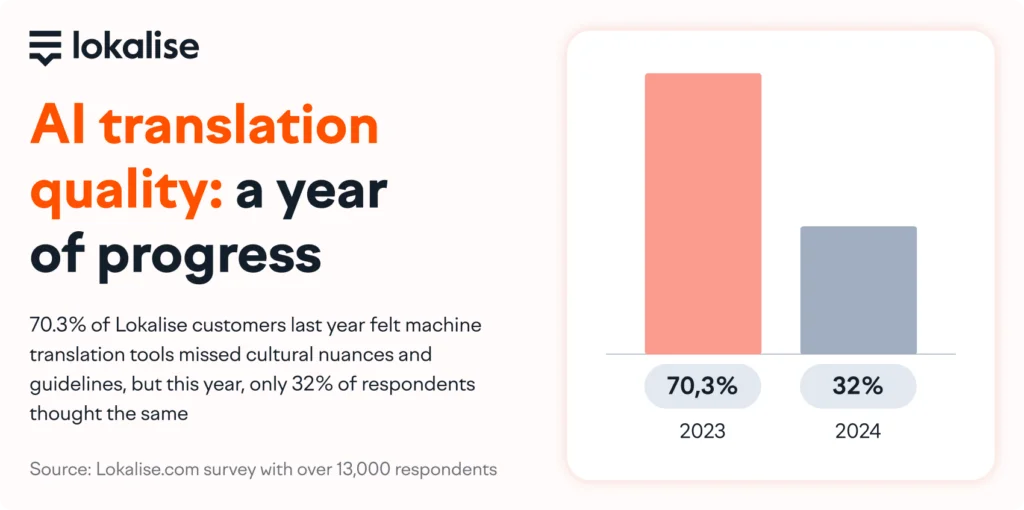
- 70.3% of respondents believed that machine translation tools often failed to capture nuances and cultural references in text
- Users frequently reported frustration with literal translations that missed the mark on context and tone
- Many still relied heavily on human translators for critical or culturally sensitive content
These findings weren’t surprising. Even fluent human speakers struggle to capture subtle nuances in language. The idea that a machine could grasp these complexities seemed unlikely to many.
Fast forward to now, and the perception of ‘machine translation tools’ has changed.
Our latest survey, though smaller in scale with 800 respondents, reveals a transformation that marks a significant turning point in the translation industry:
- Only 32% of respondents believe that ‘machine translation tools’ failed to consider context and guidelines when translating
- This represents a huge 38.3% improvement in just one year
To put it another way, in 2023, seven out of ten users were dissatisfied with AI’s ability to handle nuance and cultural context. Now, more than two-thirds of users are satisfied.
🔑 Key finding
70.3% of Lokalise customers last year felt machine translation tools missed cultural nuances and guidelines, but this year, only 32% believe that ‘machine translation tools’ failed to consider context and guidelines when translating.
So, we can now clearly state that advancements in AI technology are paving the way for better translation quality at a faster rate than expected, marking a year of remarkable progress.
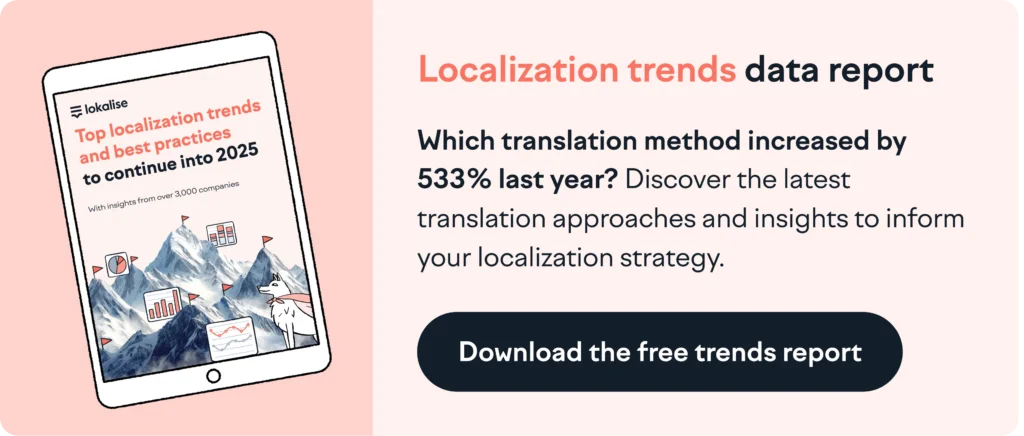
The future of translation technology
Translation technology has improved tenfold, first from its humble 250-word beginnings to the neural networks that made way for Google and DeepL, to now, with GenAI serving up translation with context and creating multilingual content from scratch.
But, the accuracy of AI translation technology remains a concern, highlighted in our survey:
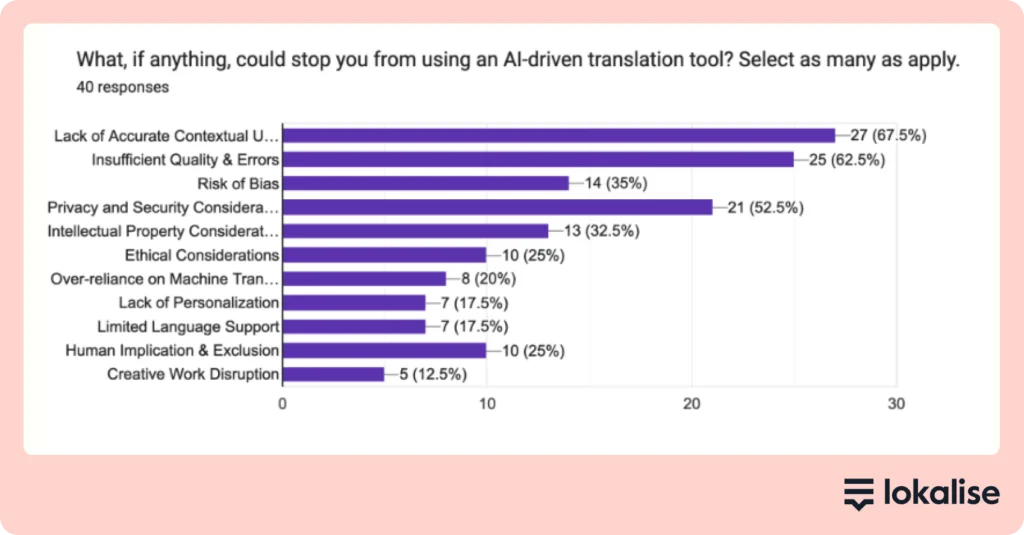
GenAI translation tools are better for some language pairs than others, down to the amount of available data that these tools use to translate and generate content. There’s also some concern about the subjectivity of these tools, with 35% of respondents worried about the risk of bias.
So, before companies can fully trust translation technology, innovators need to overcome these hurdles.
To wrap up, and to understand what we can expect from translation technology moving forward, we caught up with Adam Soltys, Senior Lead Product Manager at Lokalise, to hear his predictions on where translation technology is heading.
- Large language models will soon match professional human translation quality, especially for common language pairs. Even for high-quality translation demands, we will soon be able to delegate 90% of translations to AI
- Translation tools will face the same limitations as human translation does. They will still need context
- Providing context will be a differentiating factor in the quality of translation. But full context, not just as a style guide. GenAI translation tools will need screenshots, descriptions of UI components, cultural differences, and more, making translation management tools essential to manage all of these elements
- AI-driven translations will continue to drive down costs making localization more accessible, and offering higher ROI
- We will continue to see a high demand for personalized LLMs